Do you want to contribute by writing guest posts on this blog?
Please contact us and send us a resume of previous articles that you have written.
Unveiling the Secrets of Neural Network Learning Theoretical Foundations - Mastering the Art of Artificial Intelligence

In this digital age, the concept of Artificial Intelligence (AI) has taken the world by storm. From self-driving cars to virtual assistants, AI has revolutionized various industries. At the heart of AI lies neural networks - powerful algorithms that mimic the functioning of the human brain. But what brings these neural networks to life? The answer lies in the theoretical foundations of neural network learning.
Understanding Neural Networks
Before diving into the theoretical foundations of neural network learning, it is essential to understand what neural networks are and how they work. Neural networks are computational models composed of interconnected artificial neurons. These networks are designed to recognize patterns, make predictions, and learn from input data.
Inspired by the structure and functioning of biological brains, neural networks consist of layers of neurons, each processing and passing information to the next layer. These connections are strengthened or weakened based on the input data and desired outputs. Through a process called training, neural networks adjust these connections to improve their ability to perform specific tasks.
4.8 out of 5
Language | : | English |
File size | : | 33804 KB |
Screen Reader | : | Supported |
Print length | : | 404 pages |
The Power of Neural Network Learning
Neural network learning is the cornerstone of AI, enabling machines to learn and adapt without explicit programming. By extracting patterns and relationships from vast amounts of data, neural networks can solve complex problems and make accurate predictions.
But how do these artificial neural networks learn? The answer lies in the underlying theoretical foundations.
1. Activation Functions
Activation functions play a crucial role in determining the output of a neural network. These functions introduce non-linearities into the network, allowing it to model complex relationships between inputs and outputs. Common activation functions include the sigmoid, tanh, and ReLU functions.
By applying these activation functions to the outputs of each neuron, neural networks can capture and represent intricate patterns and nonlinear mappings.
2. Loss Functions
Loss functions are essential in neural network learning as they quantify the discrepancy between predicted outputs and actual target outputs. These functions provide a measure of how well the network is performing, allowing for the adjustment of connection strengths. Popular loss functions include mean squared error and cross-entropy loss.
By minimizing the loss function, neural networks actively learn from their mistakes, improving their predictive capabilities over time.
3. Backpropagation Algorithm
The backpropagation algorithm is a fundamental component of neural network learning. This algorithm allows for the efficient adjustment of connection weights based on the error calculated by the loss function.
During the training phase, backpropagation calculates the gradient of the loss function with respect to each connection weight. This gradient information is then used to update the connection weights, gradually minimizing the overall error.
4. Regularization Techniques
Overfitting is a common issue in neural network learning, where the model becomes overly complex and fails to generalize well to unseen data. To overcome this problem, regularization techniques are employed.
Techniques like L1 and L2 regularization impose penalties on large connection weights, discouraging overemphasis on certain features. Dropout regularization randomly sets a fraction of neuron outputs to zero during training, preventing over-reliance on specific neurons.
The Future of Neural Network Learning
Theoretical advancements in neural network learning have significantly contributed to the remarkable progress in AI. These foundations enable neural networks to learn from massive datasets, uncover hidden patterns, and make intelligent decisions.
As we delve deeper into the frontier of AI, researchers are continuously developing novel techniques and algorithms to enhance neural network learning. Recent advancements in deep learning and reinforcement learning are pushing the boundaries of what neural networks can achieve.
Deep learning focuses on training neural networks with many layers, allowing for the extraction of progressively higher-level features. This approach has led to breakthroughs in image and speech recognition, natural language processing, and more.
Reinforcement learning takes inspiration from behavioral psychology and aims to train agents to make decisions based on positive or negative feedback received from their environment. This area of research has produced impressive results in game playing and autonomous robotic control.
The future of neural network learning holds the promise of even greater advancements in AI. As these theoretical foundations continue to evolve, we can expect more sophisticated and intelligent machines that can revolutionize numerous industries, from healthcare to finance, and beyond.
The Journey Continues
Understanding the theoretical foundations of neural network learning is just the beginning of a mesmerizing journey into the world of AI. As technologies continue to evolve, so does our capacity to unravel the mysteries of the human brain and unlock the true potential of artificial intelligence.
So, embrace the power of neural network learning, and let us embark on an incredible voyage where the boundaries of human and machine intelligence blur!
4.8 out of 5
Language | : | English |
File size | : | 33804 KB |
Screen Reader | : | Supported |
Print length | : | 404 pages |
This book describes theoretical advances in the study of artificial neural networks. It explores probabilistic models of supervised learning problems, and addresses the key statistical and computational questions. Research on pattern classification with binary-output networks is surveyed, including a discussion of the relevance of the Vapnik–Chervonenkis dimension, and calculating estimates of the dimension for several neural network models. A model of classification by real-output networks is developed, and the usefulness of classification with a 'large margin' is demonstrated. The authors explain the role of scale-sensitive versions of the Vapnik–Chervonenkis dimension in large margin classification, and in real prediction. They also discuss the computational complexity of neural network learning, describing a variety of hardness results, and outlining two efficient constructive learning algorithms. The book is self-contained and is intended to be accessible to researchers and graduate students in computer science, engineering, and mathematics.
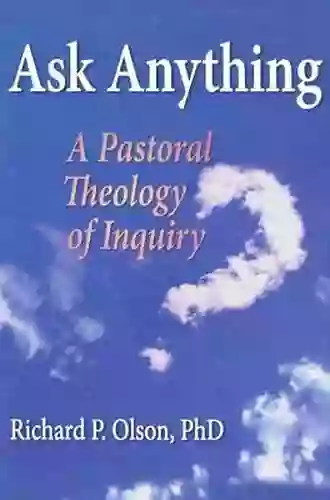

The Secrets of Chaplaincy: Unveiling the Pastoral...
Chaplaincy is a field that encompasses deep...
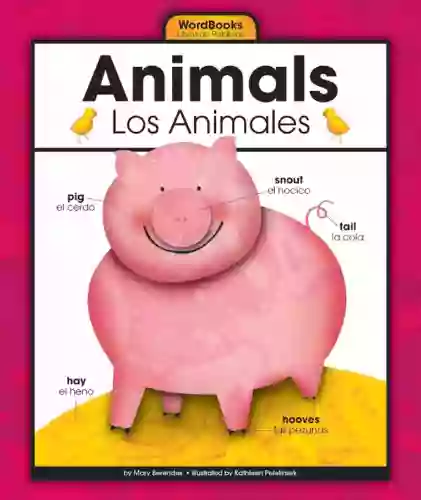

Animales Wordbooks: Libros de Palabras para los Amantes...
Si eres un amante de los animales como yo,...
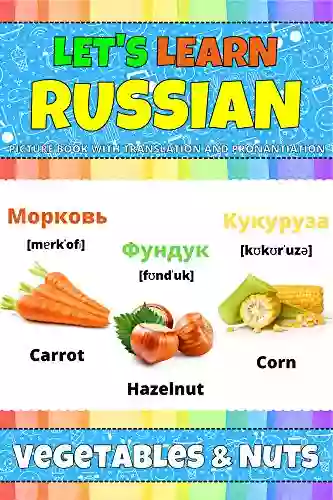

Let's Learn Russian: Unlocking the Mysteries of the...
Are you ready to embark...
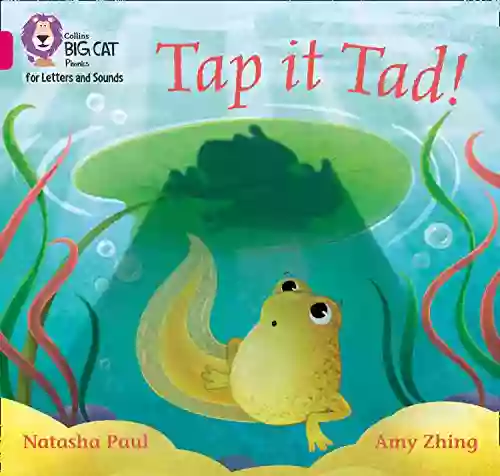

The Incredible Adventures of Tap It Tad: Collins Big Cat...
Welcome to the enchanting world of...
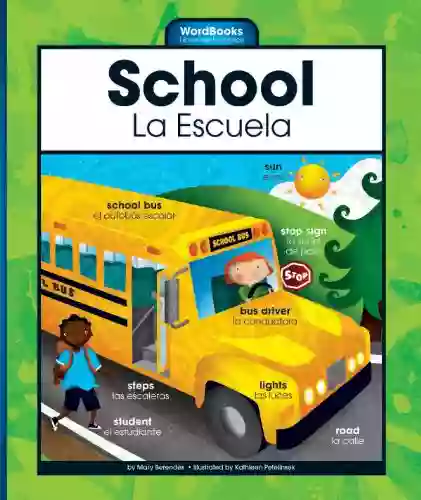

Schoolla Escuela Wordbookslibros De Palabras - Unlocking...
Growing up, one of the most significant...
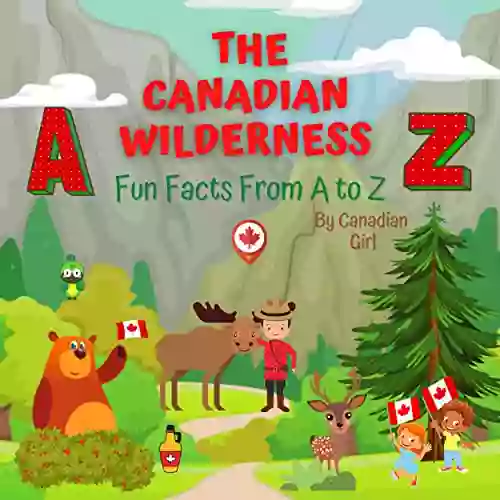

15 Exciting Fun Facts About Canada for Curious Kids
Canada, the second-largest...
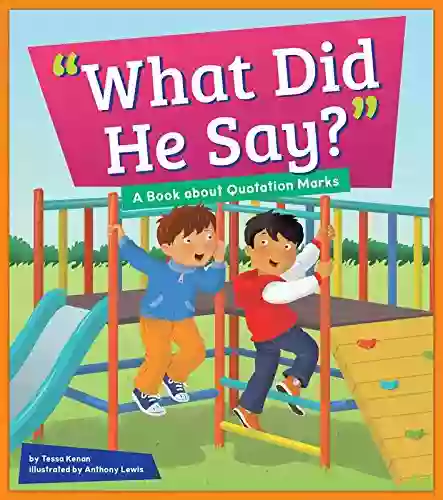

What Did He Say? Unraveling the Mystery Behind His Words
Have you ever found yourself struggling to...
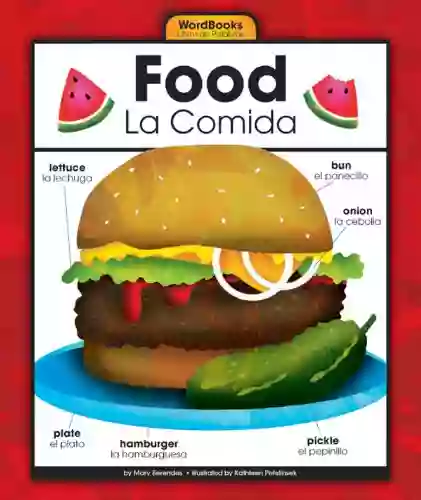

A Delicious Journey through Foodla Comida Wordbookslibros...
Welcome to the world of Foodla Comida...
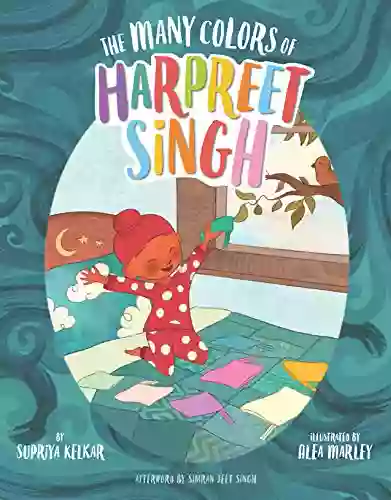

The Many Colors of Harpreet Singh: Embracing...
In a world that often...
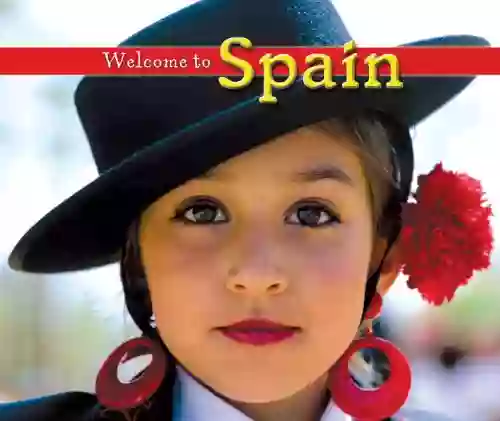

Welcome To Spain Welcome To The World 1259
Welcome to Spain, a country that captivates...
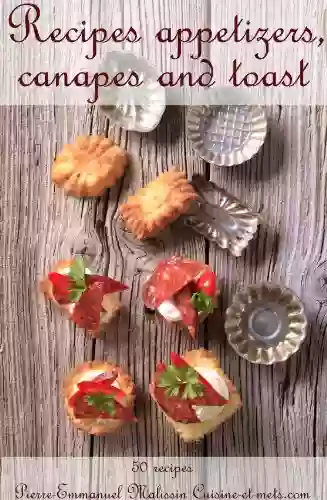

Amazing Recipes for Appetizers, Canapes, and Toast: The...
When it comes to entertaining guests or...
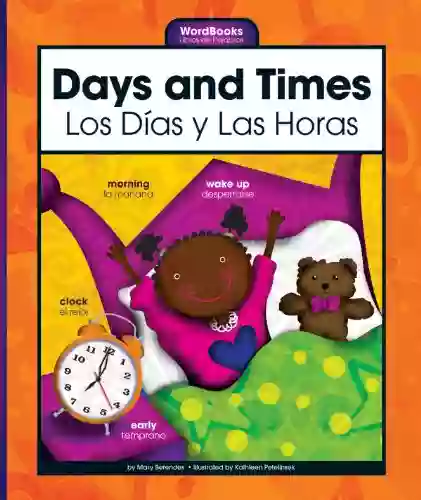

Days And Times Wordbooks: The Ultimate Guide to Mastering...
In the realm of language learning,...
Light bulbAdvertise smarter! Our strategic ad space ensures maximum exposure. Reserve your spot today!
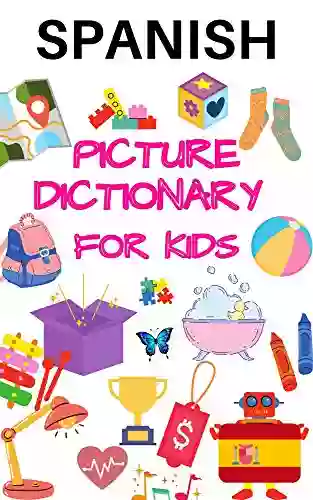





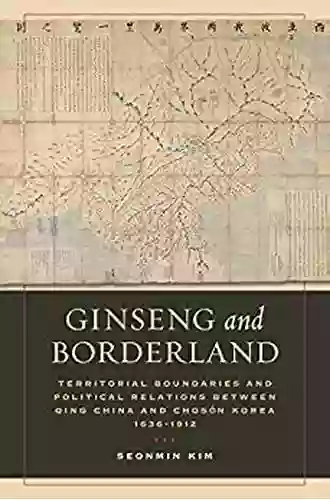

- Louis HayesFollow ·7.6k
- Hudson HayesFollow ·11.4k
- Grant HayesFollow ·12.9k
- Jonathan HayesFollow ·8.5k
- Edward ReedFollow ·18.1k
- Rick NelsonFollow ·12.2k
- Finn CoxFollow ·17k
- Henry GreenFollow ·10.3k